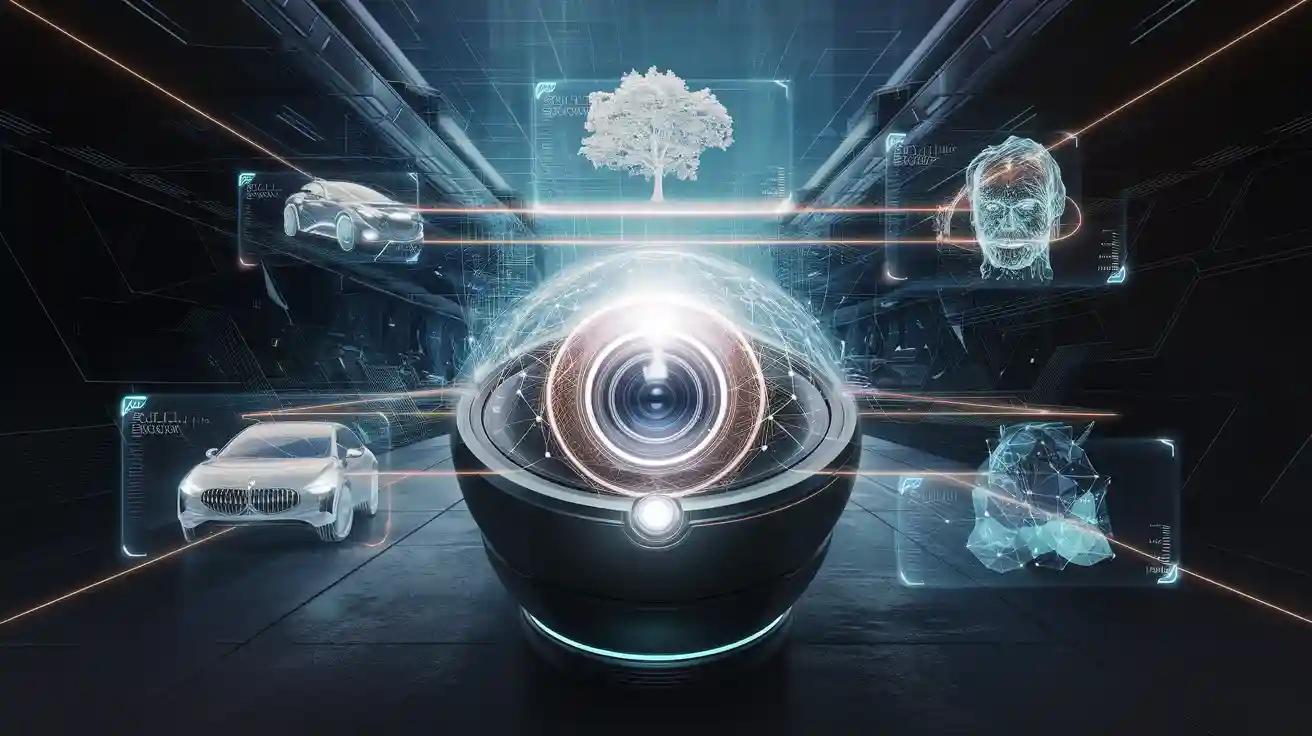
Imagine a world where machines see as clearly as you do—maybe even better. In 2025, this world becomes reality. Thanks to groundbreaking image recognition trends, industries are rewriting their playbooks. Factories now spot flaws in products faster than a hawk eyeing its prey. Hospitals diagnose diseases with precision that feels almost magical. These advancements not only slash costs but also boost quality. You’ll witness fewer errors and smarter systems everywhere. The future isn’t just knocking; it’s kicking the door wide open.
Key Takeaways
-
Vision Transformers are changing image recognition by looking at pictures as a whole. This makes results more accurate and faster.
-
Multimodal learning mixes data from different places. It helps doctors find problems and makes shopping more personal.
-
Self-supervised learning helps AI learn from data without labels. This makes it more accurate and needs less human work.
-
Edge computing lets devices recognize images right away. It works faster and better for things like security and tracking items.
-
Generative AI makes super-realistic pictures. This improves training data, removes mistakes, and makes systems smarter.
Advancements in AI for Image Recognition
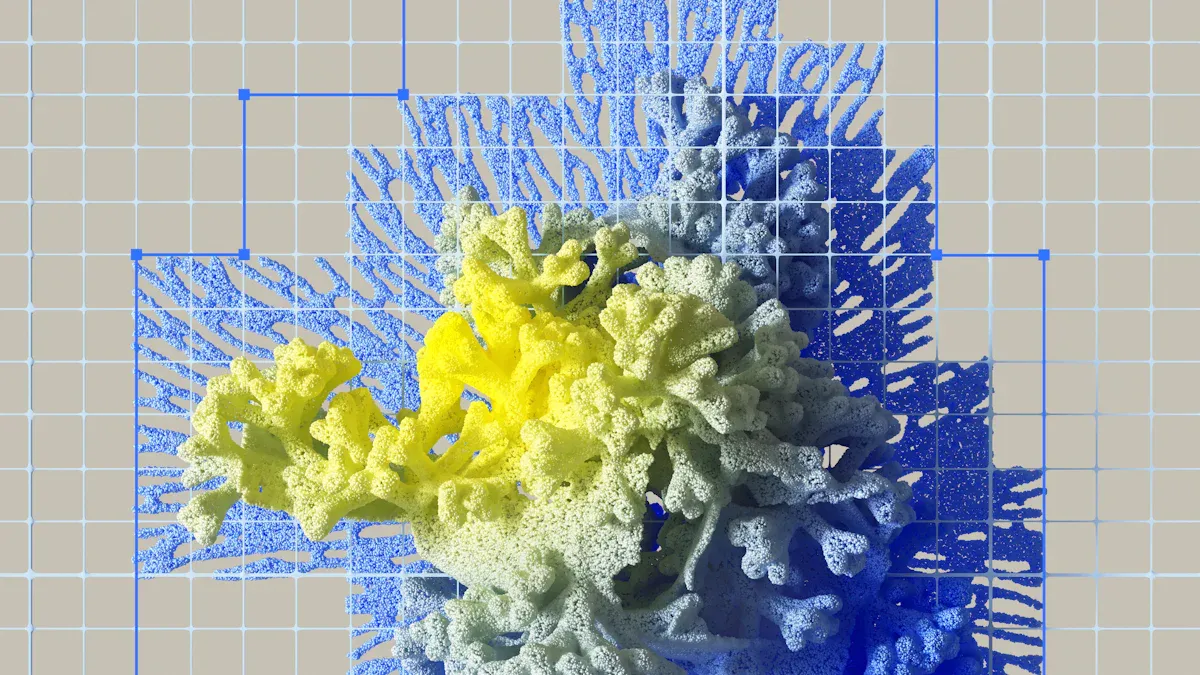
Vision Transformers in computer vision
You’ve probably heard of Convolutional Neural Networks (CNNs), the long-time champions of computer vision. But in 2025, Vision Transformers (ViTs) are stealing the spotlight. These AI-driven image recognition models are revolutionizing how machines interpret visual data. Unlike CNNs, which rely on localized filters, Vision Transformers process images holistically, capturing global context with unmatched precision.
Imagine a face recognition system that doesn’t flinch at occlusions like sunglasses or masks. Vision Transformers excel in such scenarios, delivering higher accuracy and faster inference speeds. They also consume less memory, making them ideal for resource-constrained environments. Businesses are already leveraging this technology to enhance image classification tasks, from identifying defective products to improving augmented reality applications.
Tip: Vision Transformers are not just smarter; they’re faster. If you’re looking to upgrade your computer vision systems, this is the trend to watch.
Multimodal learning for image recognition
Why settle for one type of data when you can combine multiple? Multimodal learning is the secret sauce behind the next wave of advancements in AI and deep learning models. By integrating data from various sources—images, text, and even audio—multimodal systems deliver richer insights and more accurate predictions.
Take healthcare, for example. Multimodal learning enables AI to analyze medical images alongside patient records, improving diagnosis accuracy. In retail, it powers personalized shopping experiences by combining visual cues with customer preferences. A recent study even showed how multimodal learning boosts survival predictions for cancer patients by using data from multiple cancer types.
Application |
Benefit |
---|---|
Healthcare |
Enhanced diagnosis accuracy |
Retail |
Personalized shopping |
Security |
Context-aware threat detection |
This approach isn’t just about better image recognition; it’s about smarter systems that understand the world more like you do.
Self-supervised learning advancements
Teaching AI without labeled data sounds like a dream, right? Self-supervised learning makes it a reality. This technique allows AI to learn from unlabeled data, reducing the need for costly and time-consuming manual annotations.
In 2025, self-supervised models are setting new benchmarks in image classification. They outperform traditional supervised methods, achieving top-tier accuracy on datasets like ImageNet. In medical imaging, self-supervised pre-training has led to a 7–10% increase in tumor segmentation accuracy. These advancements are reshaping industries, from healthcare to entertainment, where AI-driven image recognition is creating lifelike visuals for augmented reality applications.
Note: Self-supervised learning isn’t just a trend; it’s a game-changer. If you’re in the business of artificial intelligence, this is the innovation you can’t ignore.
Real-Time Object Detection on Edge Devices
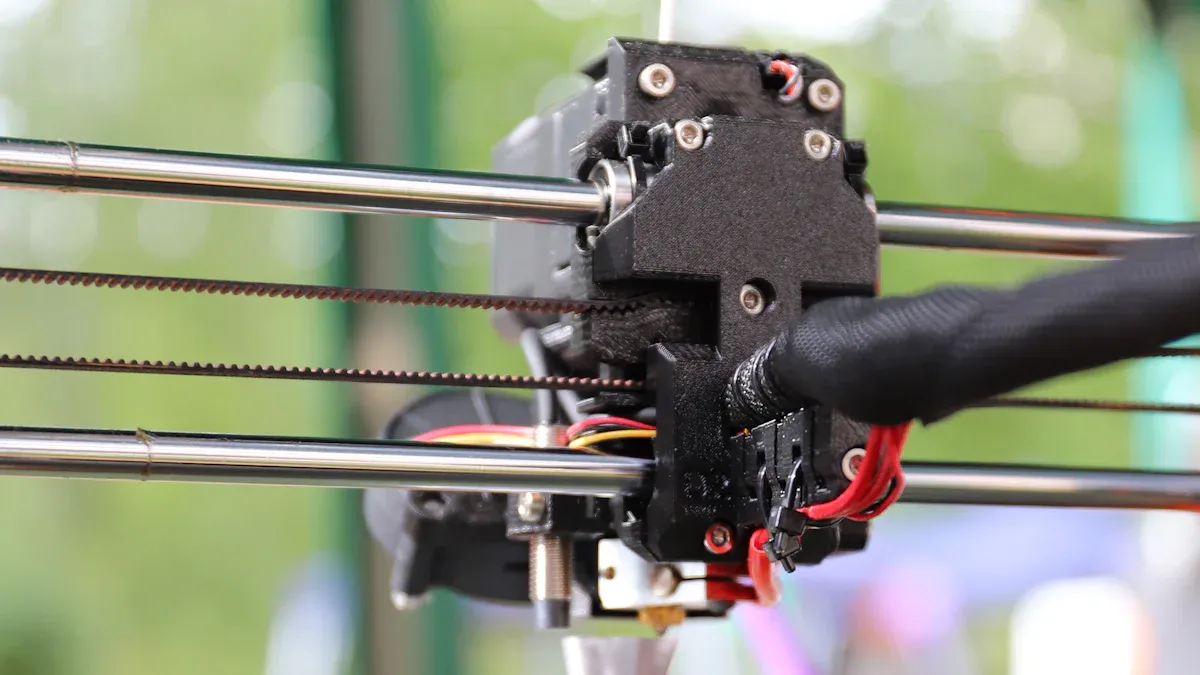
Efficiency in edge computing for image recognition
Imagine a construction site buzzing with activity. Workers move materials, machines hum, and decisions need to happen fast. This is where edge computing shines. By processing data locally, edge devices eliminate the need to send information to distant servers. You get instant results, whether it’s identifying faulty equipment or tracking inventory.
Take NEC’s edge computing solutions, for example. They’ve developed systems like walkthrough face recognition and human behavior analysis. These tools process images right at the edge layer, delivering real-time image recognition without breaking a sweat. In construction management, edge computing frameworks have achieved high accuracy in image classification tasks while reducing model sizes by 80%.
Contribution |
الوصف |
---|---|
Edge Computing Framework |
Optimized real-time image classification on construction sites. |
Multimodal Synchronization |
Enhanced situational awareness by integrating visual, audio, and text data. |
Practical Examples |
Demonstrated zero latency in material differentiation tasks. |
Edge computing doesn’t just save time; it transforms how industries handle image recognition challenges.
Reducing latency in object detection
Latency is the enemy of real-time applications. Imagine waiting seconds for your smart camera to detect an object—it’s frustrating, right? Researchers are tackling this problem head-on. Studies show that models like YOLOv4-Tiny and SSD MobileNet V2 achieve lightning-fast inference times on devices like Google Coral Dev Boards and NVIDIA Jetson Nano.
Study |
Models Evaluated |
Metrics |
Devices |
---|---|---|---|
Cantero et al. |
SSD, CenterNet, EfficientDet |
Warm-up time, Inference time |
NXP i-MX8M-PLUS, Google Coral |
Kang and Somtham |
YOLOv4-Tiny, SSD MobileNet V2 |
Detection accuracy, Latency |
NVIDIA Jetson Nano, Jetson Xavier NX |
Bulut et al. |
YOLOv5-Nano, YOLOX-Tiny, YOLOv7-Tiny |
Average precision, Latency |
NVIDIA Jetson Nano |
These benchmarks prove that object detection and tracking can happen in real-time, even on resource-constrained devices. Faster detection means smarter systems, whether it’s for security cameras or autonomous drones.
Energy-efficient models for IoT and mobile devices
Your smartphone doesn’t need to drain its battery to perform object detection. Energy-efficient models are rewriting the rules for IoT and mobile devices. Researchers have developed algorithms that optimize energy consumption based on WiFi signal strength and packet types. This means your devices can perform real-time video analysis without guzzling power.
Evidence Description |
Key Insights |
---|---|
Impact of WiFi Signal Strength |
Varying signal strengths affect energy consumption, enabling optimization. |
Energy Consumption Modeling |
Models correlate energy use with signal strength and packet types. |
Experimental Approach |
Demonstrated energy-efficient performance under normal WiFi conditions. |
These advancements make energy-efficient image recognition a reality. Whether it’s a smart home device or a wearable gadget, you’ll enjoy longer battery life without sacrificing performance.
Generative AI and Image Synthesis Trends
GANs for realistic image generation
Imagine a world where computers create images so lifelike that even experts can’t tell the difference. That’s the magic of Generative Adversarial Networks (GANs). These AI models are redefining image recognition by generating hyper-realistic visuals. From medical imaging to entertainment, GANs are everywhere.
Take healthcare, for instance. GANs can synthesize T1-weighted brain MRIs or high-resolution skin lesion images that look as real as the originals. In fact, trained radiologists often struggle to distinguish between real and GAN-generated lung cancer nodule images.
Study Description |
Findings |
---|---|
GAN for brain MRIs |
Generated images comparable in quality to real ones |
High-resolution skin lesion images |
Experts couldn’t reliably tell real from fake |
GAN-generated lung cancer nodule images |
Nearly indistinguishable from real images, even by trained radiologists |
These advancements don’t just stop at creating images. They also enhance computer vision systems by providing synthetic data for training, making them smarter and more adaptable.
AI-driven content creation in entertainment
Lights, camera, AI! The entertainment industry is embracing AI-driven content creation like never before. You’ve got AI writing scripts, composing music, and even creating virtual artists. This isn’t just cool—it’s revolutionary.
AI tools are slashing production times by 40% and cutting costs by 35%. They’re also boosting audience engagement by 28%. In North America, where AI dominates 33.6% of the market, filmmakers are using it for live sports broadcasting and multilingual content production.
Application Area |
Impact Metrics |
---|---|
Film and Television |
Revolutionizing scriptwriting and post-production workflows |
Music |
AI for composition and creating virtual artists |
Publishing |
Content generation and audience analysis |
With AI, entertainment becomes more personalized. Imagine watching a movie tailored to your preferences or listening to a song composed just for you. The possibilities are endless.
Synthetic data for enhanced image recognition
Collecting real-world data is expensive and time-consuming. Synthetic data swoops in to save the day. By using GANs and diffusion models, you can create diverse datasets that improve image recognition accuracy.
Synthetic data doesn’t just fill gaps in datasets. It reduces biases and enhances computer vision systems. For example:
-
It creates diverse scenarios for object detection.
-
It cuts down the time and cost of data collection.
A study titled Diversify, Don’t Fine-Tune showed that synthetic data boosts model performance and generalization. With this approach, you can train smarter systems faster and cheaper.
Tip: Synthetic data is the secret weapon for scaling up your image recognition projects.
Applications of Image Recognition Across Industries
Healthcare: Revolutionizing medical imaging
Imagine walking into a hospital where AI-powered systems analyze your medical images faster than you can say “X-ray.” That’s the magic of image recognition in healthcare. These systems don’t just look at images; they see patterns, detect anomalies, and even predict diseases before symptoms appear.
AI-driven image recognition is transforming medical imaging in ways that feel straight out of a sci-fi movie. Early disease detection is now a reality. AI systems can analyze chest X-rays and identify early-stage lung cancer with remarkable accuracy. A clinical study revealed that AI reduced interpretation delivery times for chest X-rays from 11.2 days to just 2.7 days. That’s not just faster—it’s life-saving.
Advancement |
Impact |
---|---|
Early Disease Detection |
AI systems analyze images quickly, aiding in identifying early-stage diseases. |
Image Segmentation |
AI accurately delineates structures like tumors, enhancing treatment planning. |
Personalized Medicine |
AI generates patient-specific insights for tailored treatment plans. |
Image-Guided Interventions |
AI provides augmented visualization and decision support during surgeries. |
Radiology Workflow Optimization |
AI reduces interpretation delivery times significantly, improving patient care standards. |
You’ll also find AI enhancing image segmentation. It can outline tumors with precision, helping doctors plan treatments more effectively. Personalized medicine is another game-changer. AI combines image data with patient records to create tailored treatment plans. Whether it’s guiding surgeons during complex procedures or optimizing radiology workflows, image recognition is revolutionizing healthcare one pixel at a time.
Fun Fact: AI systems in healthcare are so advanced, they can even predict the likelihood of certain diseases based on subtle patterns in medical images. It’s like having a crystal ball for your health!
Retail: Enhancing personalized shopping
Shopping just got smarter. Thanks to image recognition, your favorite stores now know what you want before you do. AI systems analyze your gestures, movements, and even micro-expressions to create a shopping experience that feels tailor-made.
Visual search is one of the coolest applications. Snap a picture of a product you love, and AI will find similar items in seconds. Retail giants like Target have already integrated this technology, making it easier for you to discover and purchase products.
Evidence Type |
الوصف |
---|---|
Gesture and Movement Tracking |
Captures customer interactions to provide insights into preferences and behaviors. |
Personalized Experiences |
Data analysis allows for tailored shopping experiences that exceed general expectations. |
Emotional Insight |
Micro-expression analysis captures subtle facial movements to gauge shopper satisfaction and emotional responses. |
Foot Traffic Analysis |
Tracking customer movements helps retailers understand navigation patterns and optimize store layouts accordingly. |
Dwell Time Understanding |
Analyzing how long customers spend in areas informs product placement and promotional strategies. |
AI doesn’t stop there. It tracks foot traffic to optimize store layouts and analyzes dwell time to improve product placement. Imagine walking into a store where every aisle feels like it was designed just for you. That’s the power of image recognition in retail.
Pro Tip: Next time you shop online, try using visual search tools. They’re not just convenient—they’re addictive!
Security: Advanced threat detection systems
Security has leveled up, and it’s all thanks to image recognition. AI-powered systems now detect threats faster and more accurately than ever before. Whether it’s identifying suspicious objects in a crowded airport or recognizing faces in a sea of people, these systems are redefining safety.
AI excels at real-time object detection. It can spot unattended bags, weapons, or even unusual behavior in seconds. This isn’t just about catching bad guys—it’s about preventing incidents before they happen.
Industry |
Market Size (2024) |
Market Size (2025) |
CAGR (%) |
---|---|---|---|
Security |
N/A |
N/A |
N/A |
Facial recognition is another game-changer. Airports, stadiums, and even schools are using it to enhance security. These systems can identify individuals on watchlists with incredible accuracy, ensuring public safety without causing delays.
Did You Know? The image recognition market is expected to grow from $53.4 billion in 2024 to $61.97 billion in 2025, driven by advancements in AI and computer vision.
From healthcare to retail to security, image recognition is reshaping industries in ways that were unimaginable just a few years ago. The trends in 2025 are not just about technology—they’re about creating smarter, safer, and more personalized experiences for everyone.
Entertainment: Immersive gaming and media experiences
Imagine stepping into a game world so vivid, you forget it’s not real. That’s the magic of immersive gaming in 2025. Image recognition technology is the secret sauce behind this transformation. It’s not just about better graphics; it’s about creating experiences that pull you in and make you feel like you’re part of the action.
Take virtual reality (VR), for example. Developers are using image recognition to enhance spatial presence—the sensation of being inside the game world. You don’t just see the environment; you feel it. When you navigate through a VR scene, the system tracks your movements and adjusts the visuals in real time. This doesn’t just make the game look good; it makes you believe you’re actually there.
Fun Fact: Immersion isn’t just about visuals. It’s about how the game interacts with you, making every decision feel meaningful.
How does it work?
Researchers have discovered fascinating ways to boost immersion:
-
Spatial presence: You feel like you’re physically located in the game world.
-
Role identification: You don’t just play a character; you become the character.
-
Decision-making influence: Immersion shapes how you think and act within the game.
A study even found that active navigation in VR enhances feelings of presence and role identification. While it doesn’t make the visuals richer, it makes the experience more personal and engaging.
Finding |
الوصف |
---|---|
Additive effects of VR and active navigation |
Active navigation boosts feelings of presence and role identification after perceiving the scene. |
Beyond gaming
Image recognition isn’t just revolutionizing games; it’s reshaping media experiences too. Imagine watching a movie where the characters react to your emotions. AI-powered systems analyze your facial expressions and adjust the storyline to match your mood. Feeling adventurous? The plot takes a thrilling turn. Feeling sentimental? Get ready for a heartwarming twist.
Streaming platforms are also jumping on the bandwagon. They’re using image recognition to recommend content based on your viewing habits. It’s like having a personal curator who knows exactly what you want to watch.
Pro Tip: Next time you play a VR game or watch a movie, pay attention to how it adapts to you. That’s image recognition working its magic!
The future of immersive experiences
The possibilities are endless. Developers are exploring ways to combine VR with augmented reality (AR) for hybrid experiences. Imagine playing a game where the virtual world blends seamlessly with your living room. Or watching a concert where holographic performers interact with the audience.
Image recognition is the driving force behind these innovations. It’s not just making entertainment more fun; it’s making it unforgettable. So, whether you’re a gamer, a movie buff, or just someone who loves cool tech, get ready for a future where entertainment feels more real than ever.
Ethics and Sustainability in Image Recognition
Addressing bias in computer vision algorithms
Imagine teaching a robot to recognize faces, but it struggles with certain skin tones or genders. That’s bias in computer vision, and it’s a big deal. You wouldn’t want an AI system misidentifying you or anyone else because of unfair algorithms, right? Researchers are tackling this issue head-on. They’re using techniques like re-sampling and adversarial debiasing to make AI fairer.
Fairness metrics, such as Disparate Impact and Demographic Parity, help measure how well these systems treat different demographics. Without these checks, the consequences can be severe. Misrecognition in facial recognition systems has already caused real-world harm. For example, biased algorithms have led to wrongful arrests and discrimination.
Tip: Always ask if your AI tools prioritize fairness. A little accountability goes a long way in building trust.
Ethical considerations in image recognition applications
AI is powerful, but with great power comes great responsibility. Ethical concerns in image recognition are more than just buzzwords—they’re essential. Case studies reveal the risks. In 2018, Amazon Rekognition misidentified 28 Congress members as criminals, disproportionately affecting people of color. Similarly, MIT’s 2019 study exposed how facial recognition systems often fail women and individuals with darker skin tones.
Regulations are stepping in to address these issues. The EU’s AI Act of 2021 is a standout example. It enforces stringent content moderation regulations for high-risk AI applications, ensuring accountability. Other frameworks, like the Council of Europe’s AI treaty, aim to create global standards. However, these efforts still need stronger enforcement to tackle challenges like privacy violations and bias.
Did You Know? IBM’s “Diversity in Faces” dataset tried to reduce bias but sparked privacy concerns. Balancing ethics and innovation isn’t easy, but it’s necessary.
Promoting sustainable AI practices
AI isn’t just about intelligence; it’s about responsibility. Training AI models, especially for image recognition, consumes massive energy. This impacts the environment. Metrics like CO2-equivalents and electricity consumption help measure this footprint. For instance, training large models like ChatGPT generates significant carbon emissions.
To combat this, researchers focus on energy-efficient practices. Metrics such as FLOPS/W evaluate hardware efficiency, while novel measures like Carburacy balance accuracy and resource costs. Universal sustainability metrics, like Recognition Efficiency, also guide eco-friendly AI development.
Metric |
الوصف |
---|---|
CO2-equivalents |
Quantifies carbon emissions from model training. |
Electricity consumption |
Measures the environmental footprint of AI systems. |
Carburacy |
Balances model accuracy with resource costs. |
Universal Sustainability Metrics |
Assesses AI model sustainability through efficiency measures. |
Pro Tip: Choose AI solutions that prioritize sustainability. Saving the planet while innovating? That’s a win-win!
Ethics and sustainability in image recognition aren’t optional—they’re essential. By addressing bias, enforcing regulations, and adopting eco-friendly practices, you can ensure AI serves everyone fairly and responsibly.
2025 is the year image recognition reshapes the world. AI systems now understand context like never before, blending human-like intuition with machine precision. Generative AI creates hyper-realistic datasets, reducing bias and improving accuracy. Industries are thriving, from healthcare to entertainment, as these trends unlock new possibilities.
Stricter regulations, like the EU’s AI Act, ensure ethical practices and transparency. You’ll see AI-human hybrid systems moderating content with unmatched efficiency. These advancements don’t just transform industries—they redefine society.
Call to Action: Embrace these innovations responsibly. The future is bright, but it’s up to you to keep it fair and sustainable.
التعليمات
What is image recognition, and why is it important?
Image recognition is the ability of machines to identify objects, people, or patterns in images. It’s important because it powers technologies like facial recognition, medical imaging, and self-driving cars, making life smarter and more efficient.
How does AI improve image recognition?
AI enhances image recognition by learning from vast datasets. It identifies patterns, improves accuracy, and adapts to new scenarios. For example, AI can detect diseases in medical scans or recognize faces in crowded spaces with incredible precision.
Can image recognition work without the internet?
Yes! Edge devices process data locally, enabling image recognition without internet access. This reduces latency and enhances privacy, making it ideal for applications like security cameras or IoT devices.
Is image recognition safe to use?
It’s generally safe, but ethical concerns like bias and privacy exist. Developers are working on fairer algorithms and stricter regulations to ensure image recognition benefits everyone responsibly.
What industries benefit the most from image recognition?
Healthcare, retail, security, and entertainment are leading the charge. From diagnosing diseases to creating immersive gaming experiences, image recognition is transforming how these industries operate.